FindingFive’s goal is to be a one-stop-shop for designing, implementing, recruiting for, and communicating online research. FindingFive offers seamless integration with Amazon’s Mechanical Turk (MTurk), so you can quickly and easily post your study to the marketplace and start collecting data directly from your FindingFive account. (To see how easy this is, check out our full instructions on launching a study on Mechanical Turk through FindingFive!) While there are many benefits to recruiting and running participants over the Internet, there are also unique challenges. In this post, we’ve compiled a list of best practices and tips to ensure a smooth experience when launching your FindingFive study on MTurk.
- How does MTurk work?
- How can I be a responsible MTurk requester?
- What can I do to optimize the quality of my data?
- How do I maximize participation in my study?
- Can I screen participants based on demographics or skill set?
- Can I run a longitudinal or multi-session study on MTurk?
How does Mechanical Turk work?
MTurk is an Amazon, Inc. marketplace where requesters post Human Intelligence Tasks (HITs) for workers to complete. To translate this into more academic (and less transactional!) terms, the requester is the researcher who is looking for participants (workers) to complete a study (HIT).
Requesters post the HIT to the marketplace with a brief description of the task, estimated time commitment, and the amount of compensation that will be paid upon successful task completion. Workers can view available tasks and sign up for those they deem worth their while.
After workers submit completed HITs, MTurk requires requesters to approve or reject each submission. However, the process of manually approving HITs is both time consuming and ethically dubious for the researcher, as rejected workers are not paid for their time. That’s why FindingFive automatically approves all HITs for you, so you can skip this step entirely and get straight to your data. Upon approval, workers are paid straight from funds loaded into the requester’s Amazon Web Services (AWS) account.
How can I be a responsible Mechanical Turk requester?
Mechanical Turk is an effective tool for research: it provides access to large participant pools, significantly accelerates data-collection, and reduces research-associated costs. Unfortunately, with its many advantages, MTurk carries a serious limitation: the platform is largely unregulated, leaving its workers – many of whom count on the platform to supplement their income – vulnerable to scams and underpayment. When choosing to use MTurk for a research study, it is therefore imperative to proactively safeguard your participants’ well-being. Consider the following points when designing your study:
- Do not ask for personally identifiable information. Requesting such information may leave participants feeling their payment rests on their willingness to divulge. It is also an explicit violation of Amazon’s user policy.
- Represent your study accurately. In all titles, descriptions, and estimates of time, make sure to avoid misrepresentations. Asking for informed consent is a crucial component of any research study. In the MTurk world, this includes giving participants a general and accurate sense of what they’re signing up for before they even click on your HIT.
- Pay your participants fairly. While a known perk of MTurk is its ability to minimize research-associated costs, MTurk workers are a notoriously underpaid group. Don’t let other MTurk listings (many of which pay mere pennies) guide your compensation rates. For guidelines, see our section below on offering fair pay.
- Never release data linked to Worker IDs. When you complete a study on MTurk, you will have access to your participants’ Worker IDs (alphanumeric codes used by Amazon to identify workers). The code itself does not contain information that would reveal a participant’s identity. However, this number can be linked to a person’s Amazon.com shopping profile. Here’s how: if a person uses the same email address to create both a Mechanical Turk account and an Amazon.com shopping profile, Amazon will assign the same code to both profiles. If that person’s Amazon.com profile is public, content on their profile is visible to anyone and may include a real name, photos, and associated product reviews. For this reason, publicly releasing any data linking your participants to their Worker IDs creates a major privacy concern.
The MTurk blog offers some helpful tips and clarifications on being a responsible requester. With these foundations covered, we can jump into more exciting content – tips on collecting great data!
What can I do to optimize the quality of my data?
Set high values for workers’ “HIT Approval Ratings” and “Number of HIT Approvals”
HIT approval ratings indicate the percent of an MTurk worker’s submissions that have been approved, rather than rejected, by requesters. A high rating means a worker’s past submissions have been consistently satisfactory. Based on feedback from requesters, Amazon found that setting an HIT Approval Rating of at least 95% and a minimum of 5000 for the # of HIT Approvals leads to a significant increase in data quality. For more selective studies, consider setting a minimum approval rating of 98%.
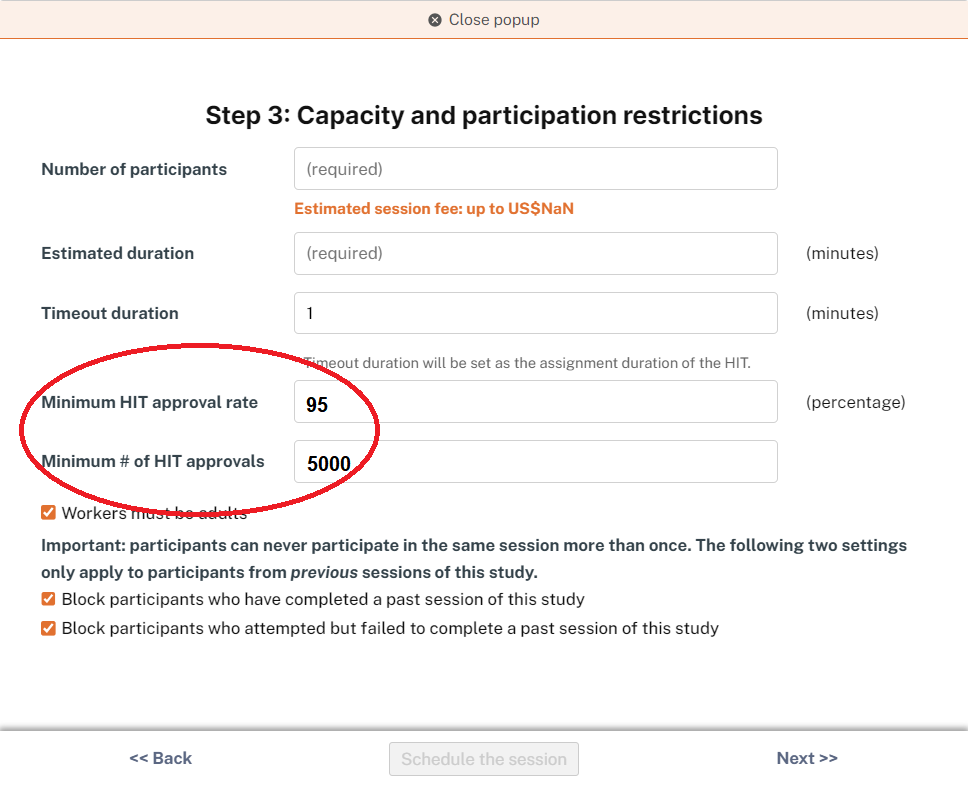
Don’t make your timeout duration too long
After accepting an HIT, participants are allotted a certain period of time within which to complete and submit their work. This period of time is called the timeout duration. When this timeout duration is too long, participants are more likely to work slowly or to leave the study partway through. In designing your task or survey, you should keep this in mind; in accordance with your study design, set a time limit that is long enough for participants to complete it comfortably, but not so long that participants are tempted to procrastinate. You can set this timeout duration straight from our Session Launch Wizard.
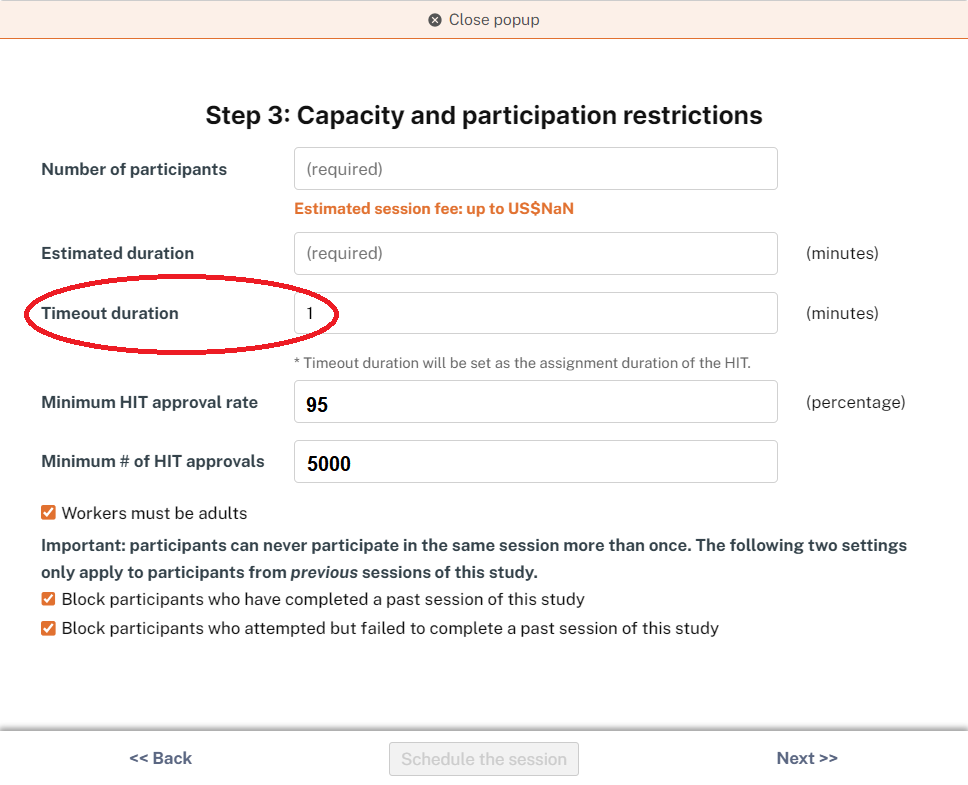
Make your instructions super clear!
Since you won’t be able to provide any on-the-spot clarification, your instructions and study-design need to be straightforward and easy to follow. Remember that your participants will be completing your study from a variety of settings and from a range of devices.
This may include a) warning participants about study requirements, such as the use of headphones for studies involving audio stimuli, b) including test rounds so that participants can practice your task before starting experimental trials, and c) displaying instructions regularly throughout the study or even on each trial, so that even participants who breeze past initial instructions can remind themselves throughout the study. Also, keep in mind issues of accessibility: for example, to accommodate participants with colorblindness, try not to make relevant stimuli green and red unless necessary for your study objectives.
Consider including catch trials
With participants completing your task on their own time and own screens, you may wonder about their attentiveness. One option to investigate attention is to make use of catch trials, also known as attention checks or instructional manipulation checks–items designed to identify participants who are merely clicking through a task without reading it carefully. Here’s an example:
Some people like to listen to music while performing household chores, like vacuuming, doing the dishes, or folding laundry. We know that not all genres of music appeal to everyone equally. To show that you’ve read this much, please ignore the question, and select only ‘Singing’ in the options below.
Please select all genres of music that you enjoy listening to.
More examples of attention checks can be found here.
While including attention checks is common practice, blanket exclusions of participants who fail a single attention check could introduce bias to your sample. This is because the likelihood of passing such checks may correlate with age, gender and race. Instead, consider using several attention checks, each with varying levels of difficulty. After your data is in, you can create a distribution of attentiveness for your participants to get a better sense of who in your sample stayed focused.
FindingFive makes it super simple to weave these catch trials into your study! Once you’ve created the templates for your instructional manipulation checks, you can make use of our catch_trials procedure to insert these items periodically throughout your study. In the final output spreadsheet, these trials will be neatly identified so you can quickly pull them out and identify the participants you want to exclude from your final dataset.
Test your study!
Try your study ahead of time, running it from start to finish. It’s helpful to ask a few people who are unfamiliar with your task to complete it – this ensures that your instructions are easy to follow and that your task runs in the way that you want it to.
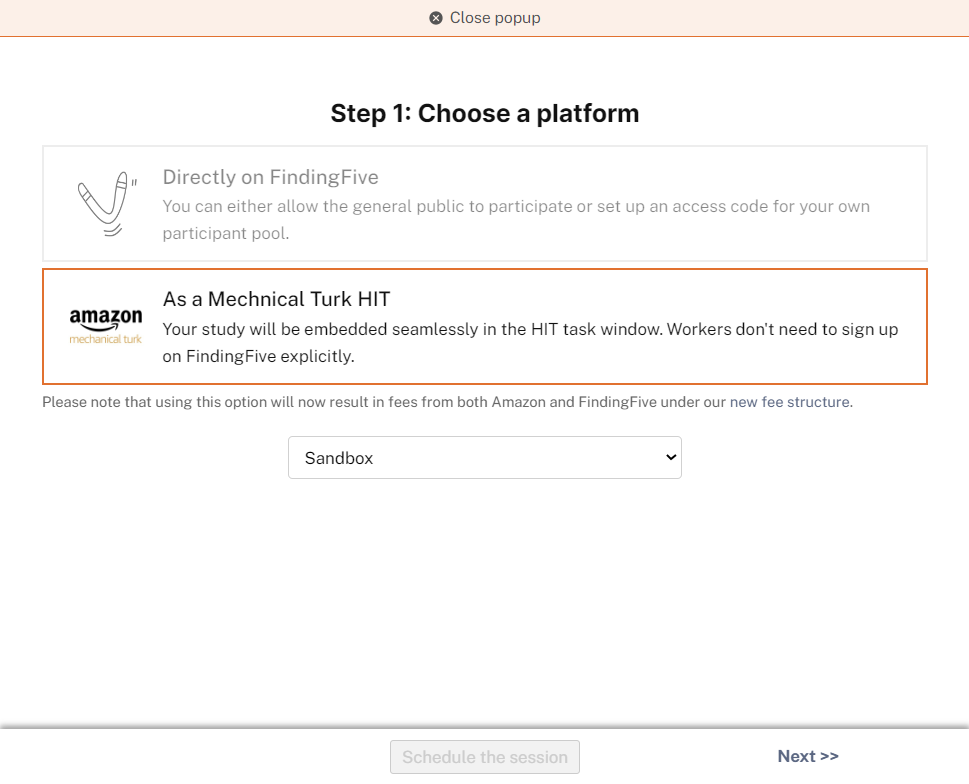
One great way to test your study is to use FindingFive’s Session Launch Wizard to launch your study straight to Mechanical Turk’s Sandbox. From the Sandbox, you are able to see your study directly on MTurk’s interface, from the point of view of your participants. Don’t worry, launching to the Sandbox does not mean that your study will be live: it will not be accessible to potential participants, and you do not need to have funds in your Amazon Web Services account for it to work.
How do I maximize participation in my study?
MTurk is a marketplace. The more appealing your study, the easier it will be to attract participants. This is especially important to consider if you have set high HIT approval minimums. Here are two straightforward strategies:
Offer fair pay!
This may seem obvious, but it bears repeating because many requesters on the Mechanical Turk platform exploit its lack of regulation. Half of MTurk’s workers make less than $5 an hour. Since many MTurk workers use the platform to supplement their income when other work is not available, it is especially important to pay your participants reasonably. In a sea of HITs paying mere pennies, a fairly paid task has a competitive edge.
It is best practice to pay your workers at least minimum wage, prorated. If a task is expected to take 10 minutes to complete, workers should be paid at least 1/6th of minimum wage. As a proxy, Amazon recommends paying $0.15- $0.20 per minute. We at FindingFive recommend going higher if you can.
In order to properly pay your participants, it is also important to accurately estimate the time required to complete your study. In the final dataset that FindingFive generates, you’ll be able to see how long participants actually spent working on your study; if your task takes most participants longer than advertised, you can send workers bonus payments to make up for their extra time.
Note that FindingFive does not directly handle payments; instead, payments are handled automatically by Amazon. Prior to posting a study, you must have loaded sufficient funds into your Amazon Web Services (AWS) account to cover payments to all participants.
Choose a catchy (but accurate) title
Your goal in naming your HIT is to catch participants’ interest while communicating useful information about what the task will entail. Avoid long, jargon-y titles. Don’t use the title you sent to the IRB!
According to this blog, workers tend to use keywords to search for HITs. So, using strategic titles will help workers locate your task. For example, if your task is quick, you might choose to put “short” in your title. If your task involves a survey, put “survey” in the title.
Can I screen participants based on demographics or skill set?
Posting a FindingFive study to Mechanical Turk using our Session Launch Wizard gives you all of the free screening functionality that is ordinarily offered by MTurk, including the ability to screen by:
- Minimum HIT Approval Rates and minimum Number of HIT Approvals
- Location of participants
- If your study’s target participants reside within the US, you may also select specific states.
- Warning: Our experiences show that Amazon’s determination of a worker’s state may be outdated and unreliable.
- Whether participants have attempted or completed another study (based on that study’s Session ID #)
(You can also learn more about how to manage MTurk workers here!)
However, you may want to run a study on a more selective population—for example, choosing participants of a certain age range or race. In these cases, here is an option (see below).
Running a Screening Survey
Another way to select a specific population for a study is to first run a brief Screening Survey through FindingFive. This process is more complicated but a good option if you require a highly specific population.
Here’s how it works:
You can create a short survey asking participants the relevant demographic questions, including a few distractor demographics so as not to reveal your variables of interest. You should include many more participants than you intend to have in your final sample, taking into account your target demographic compared to the population demographics of Mechanical Turk workers.
In the title and description of your Screening Survey, you can indicate that completion of the screening survey might make participants eligible for a longer study. You should not mention the target demographics anywhere in the title, description, or body of your Screening Survey, as workers may provide dishonest answers in the hopes of qualifying for your study.
For example, if you want to survey school teachers between the ages of 25 and 35, you could first release a short screening survey, asking workers about their ages and occupations. In this same survey, you might also ask about income level, number of household members, and race, to ensure that participants do not guess your screening criteria.
From the results of your Screening Survey, you’ll be able to identify the Worker IDs of eligible participants. When it is time to launch your main study, use Step 4.1 of our Session Launch Wizard to selectively include participants based on Worker ID. In this step, you can also choose to send these eligible workers an email notification.
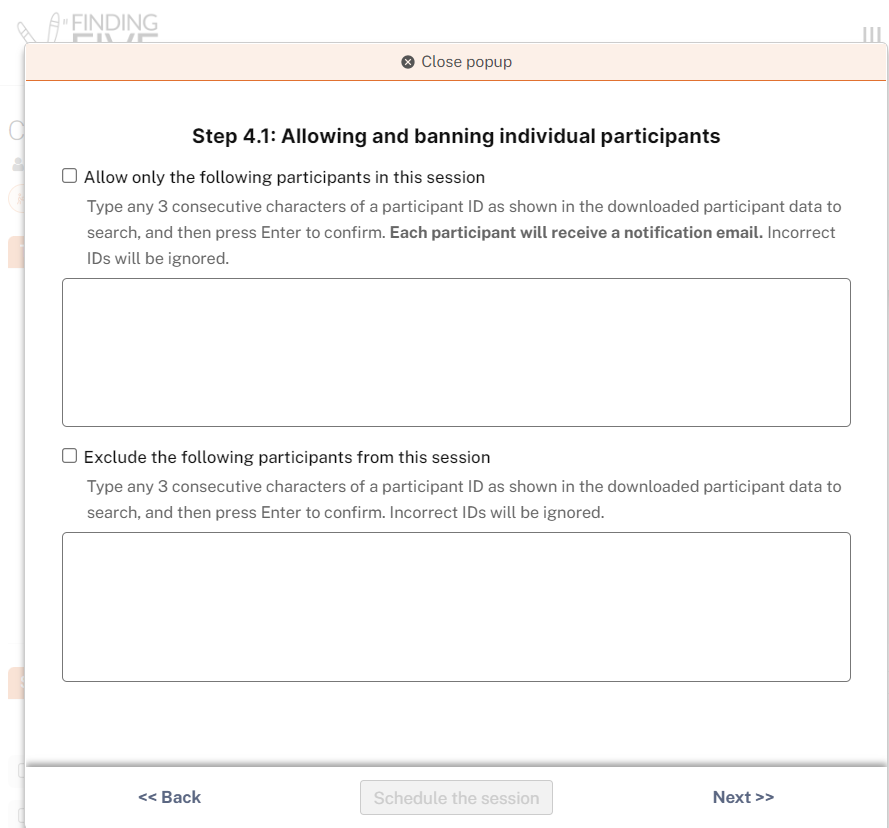
Did you know?
You can now selectively recruit participants from FindingFive’s participant pool based on basic demographics!
Can I run a longitudinal or multi-session study on MTurk?
Yes! The first step would be to post Phase 1 of your study to MTurk, including many more participants than you’d like in your final sample.
When it is time to post subsequent phases of your study, FindingFive gives you the option of allowing only workers who have completed a previous MTurk survey – based on the Session IDs found in the “Sessions” tab of the previous study – to complete the present study. That means you can select, for Phase 2, participants who have already completed Phase 1!
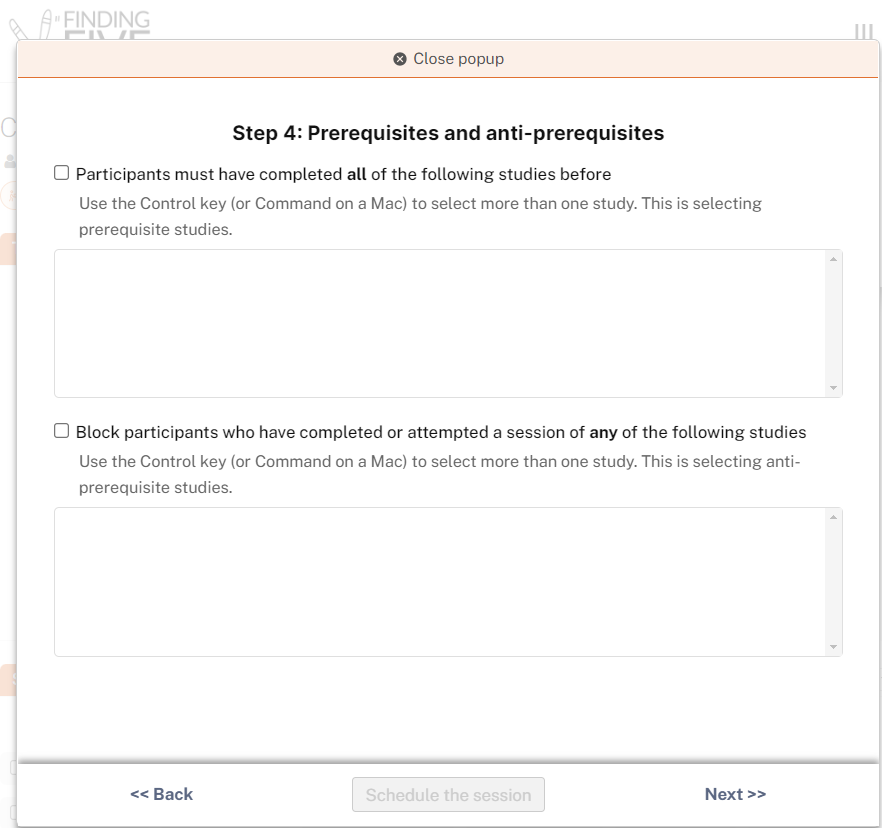
You can also use Step 4.1 of our Session Launch Wizard to include a subset of your Phase 1 participants, using Worker IDs to identify them.
Any other questions or suggestions?
We hope these tips helped you learn more about how to launch a FindingFive study to MTurk! We are always trying to improve our materials, so we welcome any additional questions or feedback you have on FindingFive’s integration with Mechanical Turk. Please email us at researcher.help@findingfive.com with comments.
Sources
Amazon Mechanical Turk. (2017, September 11). How to be a great MTurk Requester. Retrieved from https://blog.mturk.com/how-to-be-a-great-mturk-requester-3a714d7d7436
Amazon Mechanical Turk. (2016, July 13). Making HITs Available and Attractive to Workers. Retrieved from https://blog.mturk.com/making-hits-available-and-attractive-to-workers-f91613d50208
Berinsky, A.J., Margolis, M.F. and Sances, M.W. (2014), Separating the Shirkers from the Workers? Making Sure Respondents Pay Attention on Self‐Administered Surveys. American Journal of Political Science, 58: 739-753. doi:10.1111/ajps.12081
Djellel Difallah, Elena Filatova, and Panos Ipeirotis. 2018. Demographics and Dynamics of Mechanical Turk Workers. In Proceedings of WSDM 2018: The Eleventh ACM International Conference on Web Search and Data Mining, Marina Del Rey, CA, USA, February 5–9, 2018 (WSDM 2018), 9 pages.
https://doi.org/10.1145/3159652.3159661
Hitlin, Paul. ‘Research in the Crowdsourcing Age, a Case Study’ Pew Research Center. July 2016.
Available at: http://www.pewinternet.org/2016/07/11/research-in-the-crowdsourcing-age-a-case-study/
Kim In Oregon. (2015, March 13). 3 types of attention checks. Retrieved from https://mturk4academics.wordpress.com/2015/03/13/3-types-of-attention-checks/
Lease, Matthew and Hullman, Jessica and Bigham, Jeffrey and Bernstein, Michael and Kim, Juho and Lasecki, Walter and Bakhshi, Saeideh and Mitra, Tanushree and Miller, Robert, Mechanical Turk is Not Anonymous (March 6, 2013). Available at SSRN: https://ssrn.com/abstract=2228728
Oppenheimer, D. M., Meyvis, T., & Davidenko, N. (2009). Instructional manipulation checks: Detecting satisficing to increase statistical power. Journal of Experimental Social Psychology, 45(4), 867–872. https://doi.org/10.1016/j.jesp.2009.03.009
US Department of Labor, Wage and Hour Division. (Updated 2020, January 1). State Minimum Wage Laws. Retrieved from https://www.dol.gov/agencies/whd/minimum-wage/state
Comments are closed.